Preparing ONNX for use with TwinCAT Machine Learning Server
Generate interface descriptions for the PLC
To be able to use an ONNX with the FB_MlSvrPrediction in the TwinCAT PLC, interface information is required. These are generated by the TwinCAT Machine Learning Model Manager.
Information on the supported ONNX Opset and restrictions can be found here: ONNX Support.
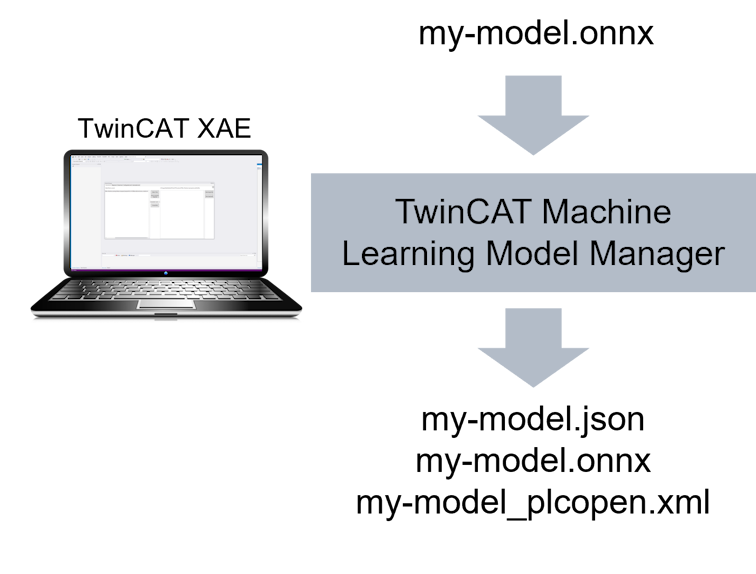
JSON file
The JSON file contains metadata required by the function block FB_MlSvrPrediction
. Users can also add their own metadata to the JSON file via the Custom Attributes. The JSON file is loaded by the function block, see Configure method. Both the JSON file and the ONNX file must be available for loading on the runtime PC, see Make model description files available on the Server Device.
PlcOpenXml
The PlcOpenXml contains the PLC type description of the input and output nodes. The automatically generated input/output structures (DUTs) reflect the input/output definition of the ONNX file provided by you. Therefore, make sure that you use meaningful names for the input and output nodes in your ONNX.
The use of these created data types is strictly required.
Sample of name generation in the PlcOpenXml:
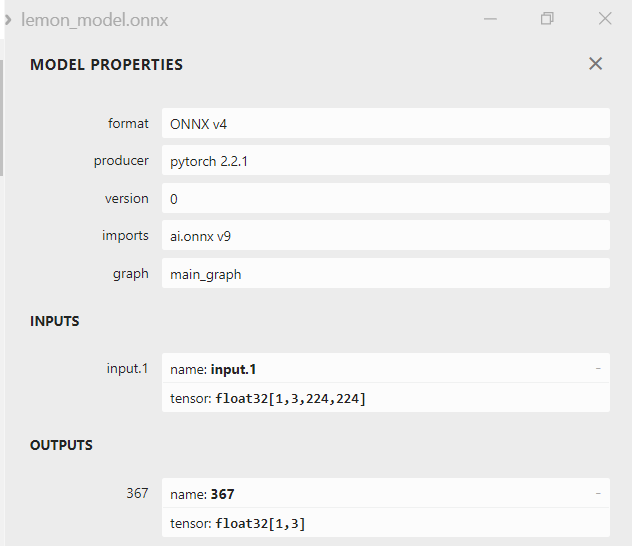
File name: lemon_model.onnx
Name Input node: input.1
Name Output node: 367
Two DUTs of type STRUCT are generated with the names ST_lemon_modelInput and ST_lemon_modelOutput. Each STRUCT has a header (do not change!) and a data area. The data area elements are named according to the input and output nodes, in the above case in_input1 and out_367. Characters not permitted in the PLC are automatically removed. If an AI model has several input or output nodes, these are each displayed as an element of the STRUCT.
Sample of the generated input data types
TYPE ST_lemon_modelInput :
STRUCT
{attribute 'hide'} _header_DO_NOT_CHANGE : ARRAY[0..63] OF SINT := [-1,-112,120,86,52,18,-1,-1,1,-1,0,0,-1,-1,-112,-112,104,-72,-97,-115,-17,4,98,-85,-50,-67,-12,-50,-6,33,-10,-11,88,80,121,12,119,-56,-24,8,110,-32,-69,113,-21,3,102,-60,64,48,9,0,0,0,0,0,0,0,0,0,0,0,0,0];
in_input1 : ARRAY[0..0,0..2,0..223,0..223] OF REAL;
END_STRUCT
END_TYPE
The header represents a hash of the shape of the input tensors (or the output tensors for the output data type) of the AI model. This ensures that the data type is used together with the correct ONNX. This also means that models with identical input and output shapes and lexicographical order carry the same hashes and therefore the data types are interoperable. This is especially important for model updates.
The byte array of the header must not be changed!
The names of the elements of the STRUCT can be adjusted if required, but not their order in the STRUCT.
Import of a PlcOpenXml in TwinCAT 3
The generated PlcOpenXml can be transferred to the PLC project in the PLC by right-clicking on a folder (e.g. DUTs) via the "Import PLCopenXML" field.
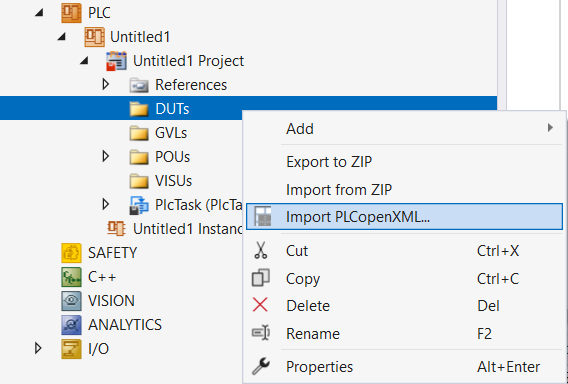
Notice | |
Verified signature: Operation of the Machine Learning Server only via generated input and output model types Note that the use of the input/output model types defined in the supplied PlcOpen file is mandatory. The types have a signature verified by the TcMlServer to ensure secure inference operations. |