ONNX export of an MLP
![]() | Download Python samples A Zip archive containing all samples can be found here: Samples of ONNX export |
MLP Regressor with PyTorch
import torch
import numpy as np
input_dim = 3
output_dim = 5
n_samples = 100
dummy_input = np.random.random((n_samples, input_dim))
dummy_output = np.random.random((n_samples, output_dim))
tensor_in = torch.FloatTensor(dummy_input)
tensor_out = torch.FloatTensor(dummy_output)
train_dataset = torch.utils.data.TensorDataset(tensor_in, tensor_out)
train_loader = torch.utils.data.DataLoader(train_dataset, shuffle=True, batch_size=32)
class MLP_Net(torch.nn.Module):
def __init__(self):
super().__init__()
self.fc1 = torch.nn.Linear(input_dim, 10)
self.fc2 = torch.nn.Linear(10, output_dim)
def forward(self, x):
x = torch.nn.functional.relu(self.fc1(x))
x = torch.sigmoid(self.fc2(x))
return x
mlp_net = MLP_Net()
optimizer = torch.optim.Adam(mlp_net.parameters(), lr =0.001)
n_epochs = 5
for epoch in range(n_epochs):
for batch in train_loader:
input, output = batch
mlp_net.zero_grad()
pred_out = mlp_net(input)
criterion = torch.nn.MSELoss()
loss = criterion(pred_out, output)
loss.backward()
optimizer.step()
onnx_file = 'pytorch_mlp.onnx'
tensor_input_size = torch.FloatTensor(np.random.random((1,input_dim))) # First dimension must be 1
torch.onnx.export(mlp_net, tensor_input_size, onnx_file, verbose=True)
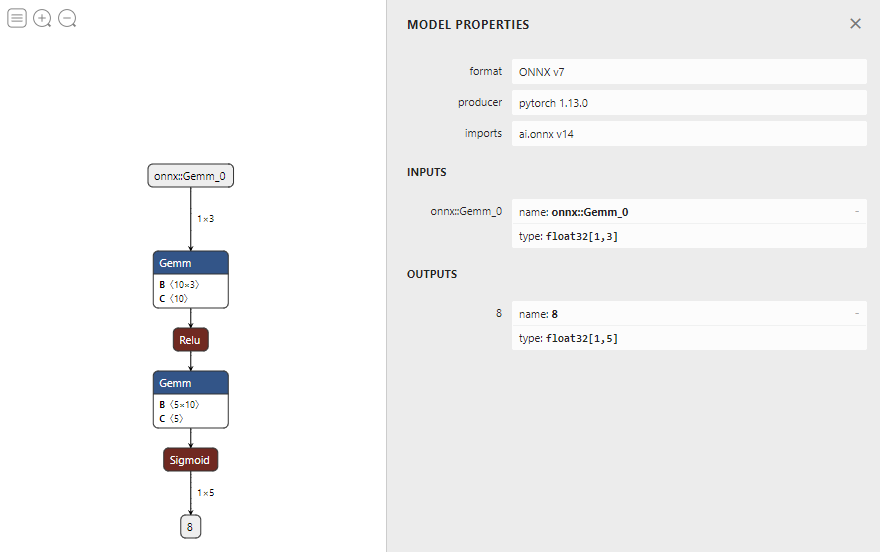
MLP Regressor with Keras
import tensorflow as tf
import numpy as np
import tf2onnx
input_dim = 3
output_dim = 5
n_samples = 100
dummy_input = np.random.random((n_samples, input_dim,))
dummy_output = np.random.random((n_samples, output_dim))
model = tf.keras.Sequential()
model.add(tf.keras.layers.Dense(5, input_shape = (input_dim,), activation=tf.keras.activations.relu, use_bias = True))
model.add(tf.keras.layers.Dense(output_dim, activation='sigmoid'))
model.build()
model.summary()
learning_rate = 0.001
loss = 'mean_squared_error'
optimizer = tf.keras.optimizers.Adamax(lr=learning_rate)
model.compile(optimizer=optimizer, loss=loss)
model.fit(x=dummy_input, y=dummy_output, batch_size=32 ,epochs=5,verbose=1, shuffle=True)
filename = 'tf_keras_mlp'
onnx_model, _ = tf2onnx.convert.from_keras(model)
with open(filename+'.onnx','wb') as f:
f.write(onnx_model.SerializeToString())
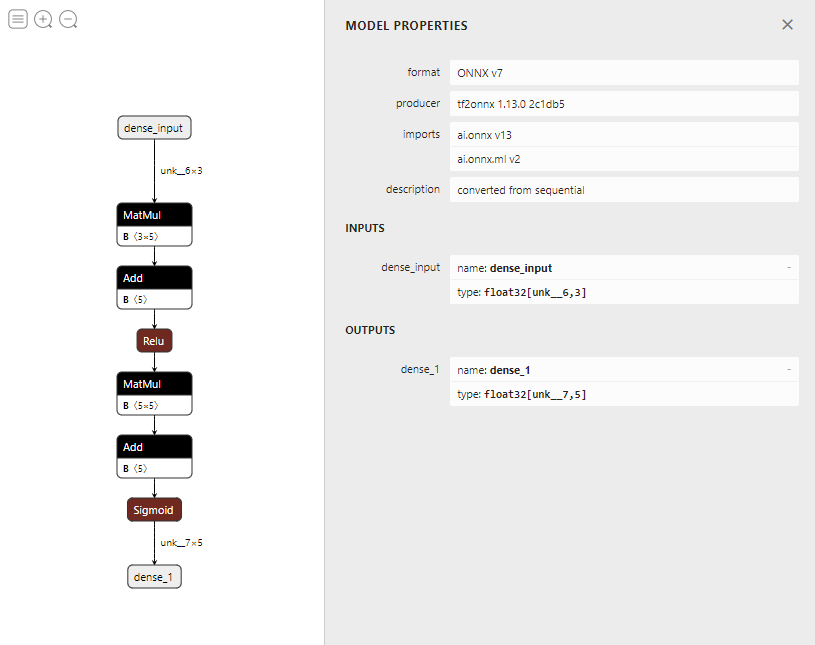
MLP Regressor with Scikit-learn
import sklearn.neural_network as skl
import numpy as np
input_dim = 3
output_dim = 5
n_samples = 100
dummy_input = np.random.random((n_samples,input_dim))
dummy_output = np.random.random((n_samples,output_dim))
model = skl.MLPRegressor(hidden_layer_sizes =(5), activation ='relu')
model.fit(dummy_input,dummy_output)
filename = 'skl_relu_reg'
from skl2onnx import convert_sklearn
from skl2onnx.common.data_types import FloatTensorType
initial_type = [('float_input',FloatTensorType([None,input_dim]))]
onx = convert_sklearn(model,initial_types=initial_type)
with open(filename+'.onnx','wb') as f:
f.write(onx.SerializeToString())
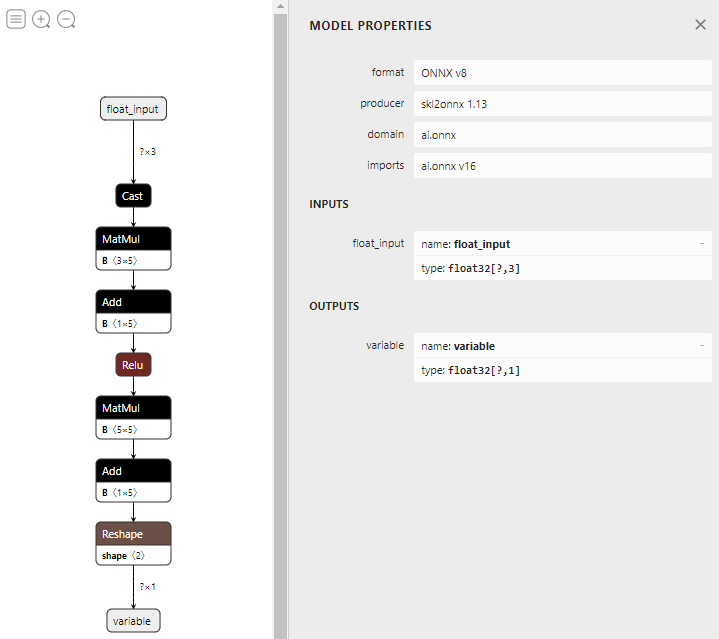
MLP Classifier with Scikit-learn
import sklearn.neural_network as skl
import numpy as np
import onnx
from skl2onnx import convert_sklearn
from skl2onnx.common.data_types import FloatTensorType
def modify_onnx_MLPClassifier(onnx_MLPCLassifier):
""" Function to modify onnx model from MLPClassifier to make it suitable for TwinCAT Machine Learning
The function removes unsupported nodes and uses the probability estimates of the classes as output for
the model.
The output of the modified onnx model is the same as the output of the predict_proba() method from
sklearn.neural_network.MLPClassifier.
To get the class as an integer output either a binarization or an argmax function must be applied to the
model output.
"""
# Delete nodes after last sigmoid/softmax layer
output_node_types = {'Sigmoid', 'Softmax'}
len_onx_init = len(onnx_MLPCLassifier.graph.node)
erased_node_inputs = []
for idx, node in enumerate(reversed(onnx_MLPCLassifier.graph.node)):
if node.op_type in output_node_types:
idx_last_node = len_onx_init - idx -1
break
else:
for idx, input in enumerate(node.input):
erased_node_inputs.append(input)
onnx_MLPCLassifier.graph.node.remove(node)
# Get output dimension and clean initializers
second_last_node = onnx_MLPCLassifier.graph.node[idx_last_node-1]
for initializer in onnx_MLPCLassifier.graph.initializer:
if initializer.name in second_last_node.input:
output_dim = initializer.dims[1]
if initializer.name in erased_node_inputs:
onnx_MLPCLassifier.graph.initializer.remove(initializer)
# Erase original outputs and create new output
for output in reversed(onnx_MLPCLassifier.graph.output):
onnx_MLPCLassifier.graph.output.remove(output)
name_new_output = "probabilities"
newOutput = onnx.helper.make_tensor_value_info(name_new_output, onnx.TensorProto.FLOAT, shape=(None, output_dim))
onnx_MLPCLassifier.graph.output.append(newOutput)
# Create new node and connect to new output
last_node_output = onnx_MLPCLassifier.graph.node[idx_last_node].output
new_node = onnx.helper.make_node("Identity", last_node_output, [name_new_output], "Identity_Out")
onnx_MLPCLassifier.graph.node.append(new_node)
return onnx_MLPCLassifier
input_dim = 3
output_dim = 2
n_samples = 100
dummy_input = np.random.random((n_samples,input_dim))
dummy_output = np.random.randint(2, size=(n_samples, output_dim))
model = skl.MLPClassifier(activation ='relu', hidden_layer_sizes=(5))
model.fit(dummy_input,dummy_output)
filename = 'skl_mlp_clf'
initial_type = [('float_input',FloatTensorType([None,input_dim]))]
onx = convert_sklearn(model,initial_types=initial_type, options={type(model): {'zipmap': False}})
onx = modify_onnx_MLPClassifier(onx)
with open(filename+'.onnx','wb') as f:
f.write(onx.SerializeToString())
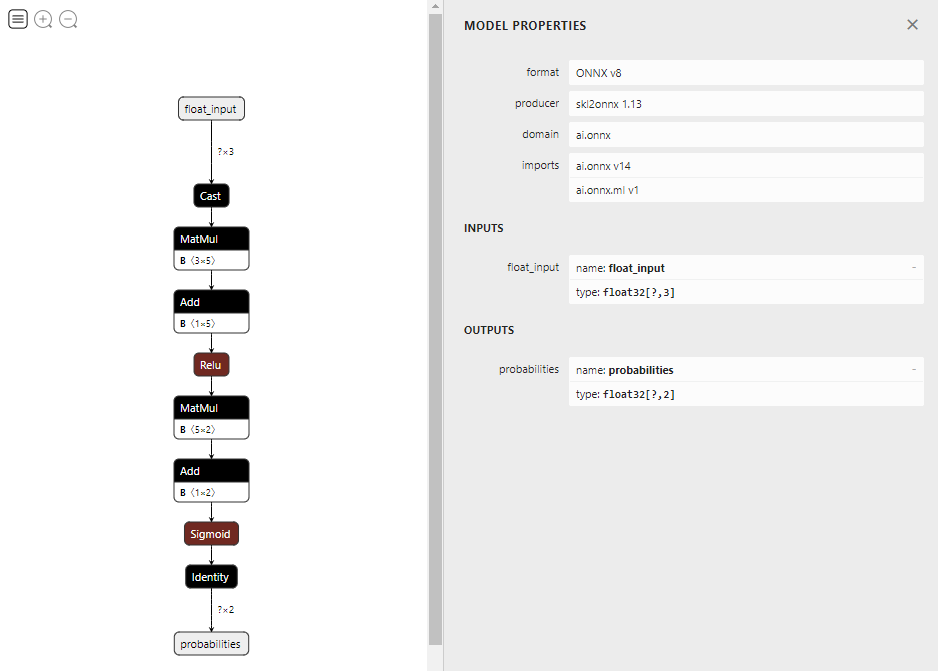
Observe the function modify_onnx_MLPClassifier. This modifies the lower part of the ONNX graph so that only operators supported by the TwinCAT Neural Network Inference Engine are used. Without this modification, the operators shown here will be generated (depending on the dimensionality of the problem). Only the area with the green border is supported.
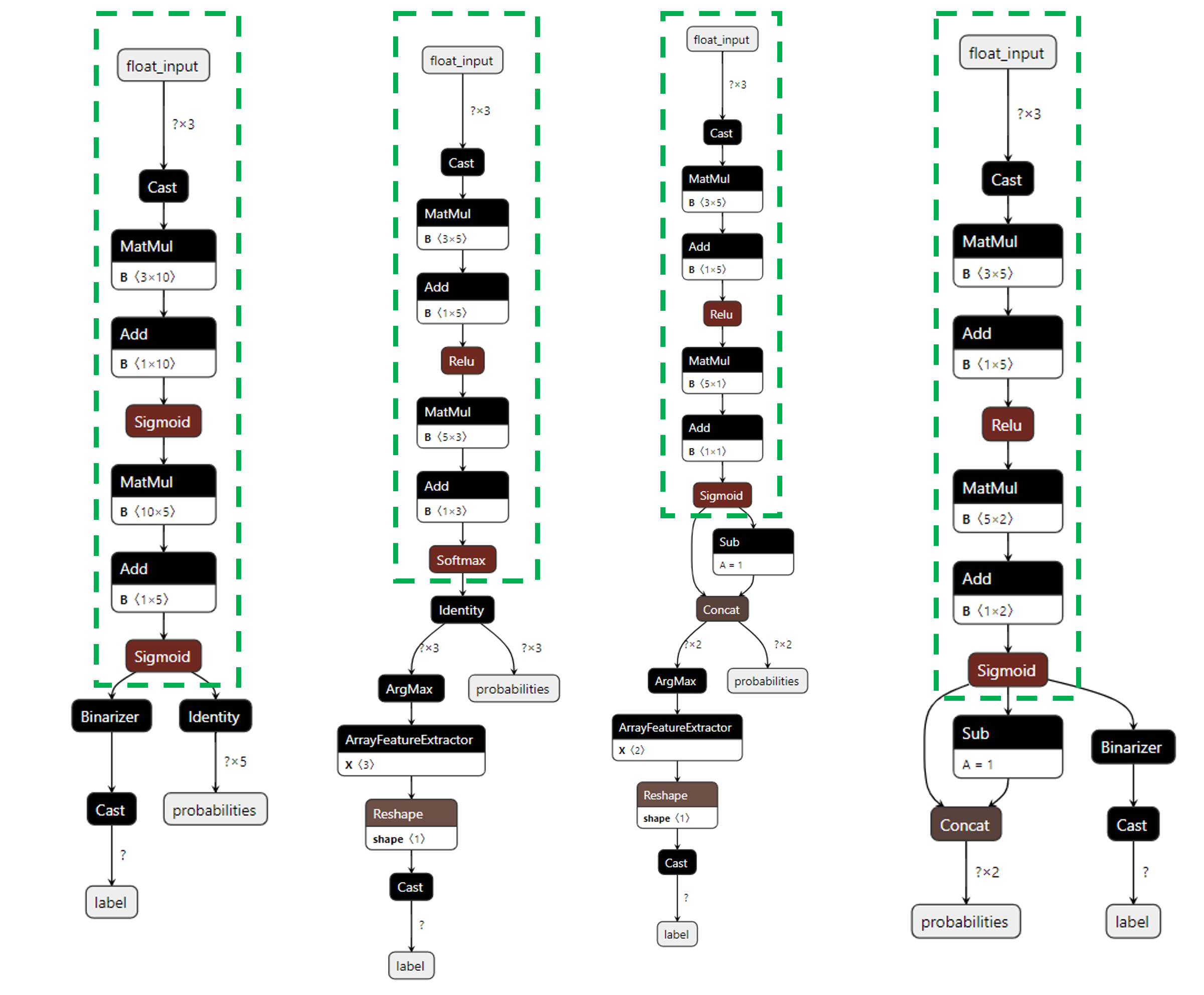